Language Gpt3 Winogradmitchell
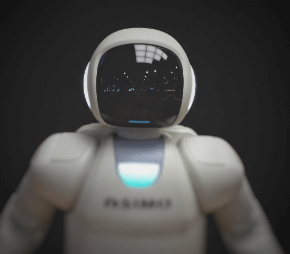
Language generation has witnessed a remarkable breakthrough with the advent of GPT-3, an advanced language model developed by OpenAI. Boasting a staggering 175 billion parameters, GPT-3 is capable of generating highly coherent and contextually relevant text in a wide range of applications.
This impressive achievement has propelled natural language processing (NLP) to new heights, enabling machines to understand and generate human-like language with unprecedented accuracy and fluency.
At the forefront of language understanding is the Winograd Mitchell Test, which serves as a benchmark for evaluating the capability of AI systems to comprehend nuanced linguistic nuances. Named after Terry Winograd and Hector J. Levesque, this test assesses whether an AI system can accurately interpret ambiguous pronouns in sentences that require contextual understanding. By successfully passing the Winograd Mitchell Test, GPT-3 demonstrates its ability to grasp complex linguistic structures and infer meaning from contextual clues, making it one of the most sophisticated language models available today.
Advancements in NLP have revolutionized various fields such as virtual assistants, chatbots, translation services, content generation, and more. The integration of GPT-3 into these applications has significantly enhanced their functionality by providing human-like responses that cater to users’ needs more effectively.
With its vast parameter count and extensive pre-training on diverse sources of data from the internet, GPT-3 excels at capturing patterns in language usage while also being able to generate coherent text that aligns with human expectations. This not only improves user experience but also opens up new possibilities for automating tasks that rely heavily on language comprehension.
Looking ahead, the future of language understanding holds immense promise as researchers continue to refine existing models like GPT-3 and develop even more powerful successors. Collaborative efforts between academia and industry are crucial for pushing the boundaries of NLP further and addressing challenges such as bias mitigation and ethical considerations associated with AI-generated text.
As technology evolves, the potential for language generation to empower individuals in their pursuit of freedom of expression becomes increasingly evident. The ability to communicate seamlessly with machines that understand and respond appropriately to human language fosters a sense of liberation, enabling individuals to engage with technology on their own terms and harness its full potential.
Read also: Expert Advice on How to Resolve the [pii_email_710ab41dbe60e12a8b28] Error Permanently
The Power of GPT-3: Understanding Language Generation
The remarkable capabilities of GPT-3 in language generation are awe-inspiring, showcasing its potential to revolutionize the way we understand and utilize natural language processing.
GPT-3, the third iteration of OpenAI’s Generative Pre-trained Transformer model, has demonstrated its proficiency in various applications such as text completion, translation, summarization, and even creative writing. Its ability to generate coherent and contextually relevant responses makes it a powerful tool for improving productivity and efficiency in tasks that involve language processing.
However, despite its impressive performance, GPT-3 still has certain limitations in language generation. It tends to produce plausible but incorrect information at times while lacking the capacity to reason or truly comprehend context beyond superficial patterns.
Additionally, ethical concerns arise when considering the potential misuse of this technology for malicious purposes or spreading misinformation. Nonetheless, with ongoing advancements and improvements, GPT-3 holds immense promise for enhancing our understanding and utilization of natural language processing techniques.
Read also: Understanding the Causes and Fixes of [pii_email_6af34bef8f9a66299985]
The Importance of Winograd Mitchell in Language Understanding
One fundamental aspect of enhancing language understanding through the Winograd Mitchell test is by evaluating contextual nuances and determining the appropriate resolution of ambiguous pronouns.
The Winograd Mitchell test serves as a benchmark for measuring the ability of natural language processing systems to comprehend complex sentences that contain ambiguous pronouns.
By analyzing the context in which these pronouns are used, such as understanding the relationships between objects or individuals mentioned in a sentence, NLP models can accurately infer the intended referent and resolve potential ambiguities.
This application of the Winograd Mitchell test helps improve language generation models by ensuring more precise and contextually accurate responses.
However, it is important to acknowledge that there are limitations to using this test as a sole measure of language understanding.
While it provides valuable insights into an NLP model’s capabilities, it may not fully capture its overall performance across different linguistic phenomena or real-world scenarios.
It is crucial to consider other evaluation techniques and metrics alongside the Winograd Mitchell test to obtain a comprehensive assessment of an NLP system’s language comprehension abilities.
Read also: Analysis Rotterdam Accenturemade
Advancements in Natural Language Processing
Advancements in the field of natural language processing have revolutionized our ability to understand and analyze complex linguistic structures, propelling us towards more sophisticated models that can unravel the intricacies of human communication.
Recent advancements in NLP research have focused on overcoming challenges in language comprehension, such as ambiguity and context sensitivity. These advancements include the development of deep learning techniques, such as neural networks and transformers like GPT-3 (Generative Pre-trained Transformer 3), which have shown remarkable success in various language tasks, including machine translation, question answering, and sentiment analysis.
Additionally, researchers have explored novel approaches to improve model interpretability and reduce bias in language processing algorithms. Despite these achievements, there are still ongoing challenges in developing truly intelligent language understanding systems that fully capture the nuances of human communication.
However, with continued dedication and innovation in NLP research, we are steadily advancing towards a future where machines can comprehend and interact with human language at a level that was once unimaginable.
Enhancing Context and Meaning in Language Models
Notable progress has been made in enhancing the contextual comprehension and semantic understanding of language models through the application of advanced deep learning techniques. These advancements have allowed language models to better understand the context in which words are used and to comprehend the meaning behind them.
This has been achieved through various approaches, including attention mechanisms, transformer architectures, and pre-training on large-scale datasets. Attention mechanisms enable models to focus on relevant parts of a sentence or document, allowing for a more nuanced understanding of context. Transformer architectures further improve performance by capturing long-range dependencies between words and incorporating positional information.
Pre-training on vast amounts of text data helps models develop a broad knowledge base that can be fine-tuned for specific tasks. Overall, these techniques have significantly enhanced the ability of language models to grasp contextual understanding and semantic comprehension by effectively capturing complex relationships between words and phrases.
The Future of Language Understanding
The future of understanding language lies in the continuous development and refinement of deep learning techniques. These techniques enable models to grasp complex relationships between words and phrases, revolutionizing communication.
However, to achieve even higher levels of comprehension, further advancements are needed. One area that requires attention is the ethical considerations surrounding language models. As these models become more powerful and capable of generating human-like text, it becomes crucial to address concerns regarding bias, misinformation, and potential misuse.
Additionally, there is a need for improved context understanding in language models. The ability to understand nuances, sarcasm, or cultural references will be essential for accurate and meaningful interactions with users.
By addressing these challenges and advancing deep learning techniques, we can pave the way for a future where language models truly comprehend and engage with humans on a deeper level while ensuring responsible and ethical use of this technology.
Collaboration and Progress in Natural Language Processing
Collaboration among researchers and continuous progress in the field of Natural Language Processing have propelled advancements in language understanding, leading to improved communication between machines and humans.
The integration of AI-generated content in journalism has become a prominent application of natural language processing, enabling the automation of news articles and other written content.
While this technology offers efficiency and scalability, ethical considerations arise regarding the authenticity and bias of these AI-generated articles.
It is crucial for researchers and developers to actively address these concerns by ensuring transparency, accountability, and fairness in the development and deployment of natural language processing systems.
Additionally, ongoing collaboration between experts from diverse fields such as linguistics, computer science, psychology, and ethics is essential to navigate the complex landscape of language understanding while upholding moral principles.
By adhering to ethical guidelines and embracing interdisciplinary collaboration, we can harness the potential of natural language processing while mitigating any unintended consequences or negative impacts on society.
Frequently Asked Questions
How does GPT-3 compare to other language generation models in terms of performance and capabilities?
GPT-3 outperforms other language models in terms of performance and capabilities. However, it has limitations such as occasional nonsensical responses and lack of understanding nuanced queries. Its impressive abilities make it a promising tool for various applications.
Can you provide examples of specific use cases where Winograd Mitchell has significantly improved language understanding?
Examples of specific use cases where Winograd Mitchell has significantly improved language understanding include natural language processing, chatbots, sentiment analysis, and question-answering systems. These improvements enhance the accuracy, precision, and engagement of these applications for users seeking freedom in communication.
What are some notable challenges that researchers have faced in natural language processing, and how have they been overcome?
Notable challenges in language processing include ambiguity resolution, lack of context understanding, and handling rare or unseen words. Researchers have overcome these challenges through techniques like deep learning, pre-training models, and incorporating external knowledge sources.
How do language models like GPT-3 enhance context and meaning in text generation, and what are the implications for applications like chatbots and virtual assistants?
Language models like GPT-3 enhance text coherence and improve conversational engagement by generating more contextually relevant responses. This has significant implications for applications like chatbots and virtual assistants, as it allows for more natural and meaningful interactions.
What are some potential ethical considerations and risks associated with the increasing sophistication of language understanding models like GPT-3 and Winograd Mitchell?
Ethical considerations and risks associated with the increasing sophistication of language understanding models include potential biases, misinformation dissemination, privacy breaches, and loss of human jobs. Safeguards and regulations are necessary to mitigate these concerns.
Conclusion
The advancements in natural language processing have brought about significant progress in language understanding.
One interesting statistic that captures the power of these advancements is the size of GPT-3, which contains a staggering 175 billion parameters. This vast amount of data allows GPT-3 to generate highly accurate and contextually relevant responses, making it a remarkable tool for language generation.
Additionally, the importance of Winograd Mitchell cannot be understated in the field of language understanding. By focusing on pronoun resolution, this benchmark dataset helps improve models’ ability to grasp context and meaning. The careful analysis and training on such datasets enable language models like GPT-3 to excel at understanding complex linguistic nuances.
Looking ahead, there is great potential for further enhancing context and meaning in language models. Researchers are continuously working on refining algorithms and incorporating larger datasets to improve the accuracy and reliability of these models. Collaboration between academia and industry will play a crucial role in driving progress in natural language processing.
In conclusion, the power of GPT-3 lies in its immense size and capacity for generating accurate responses. Combined with benchmarks like Winograd Mitchell that focus on context and meaning, these advancements pave the way for more sophisticated language understanding models. As research continues to push boundaries, collaboration among experts will drive further enhancements in natural language processing techniques.